๐ ๊ณต๋ถํ๋ ์ง์ง์ํ์นด๋ ์ฒ์์ด์ง?
์ฝ๋ก๋ ํ์ง ์๋ฐฉ์ ์ํด ์๊ณ์ด(Time-Series) ๋ฐ์ดํฐ๋ก LSTM ์์ธก ๋ชจ๋ธ๋ง๋ค๊ธฐ ๋ณธ๋ฌธ
๐ฉ๐ป ์ธ๊ณต์ง๋ฅ (ML & DL)/Serial Data
์ฝ๋ก๋ ํ์ง ์๋ฐฉ์ ์ํด ์๊ณ์ด(Time-Series) ๋ฐ์ดํฐ๋ก LSTM ์์ธก ๋ชจ๋ธ๋ง๋ค๊ธฐ
์ง์ง์ํ์นด 2022. 10. 26. 14:46728x90
๋ฐ์ํ
221026 ์์ฑ
<๋ณธ ๋ธ๋ก๊ทธ๋ data-panic ๋์ ๋ธ๋ก๊ทธ๋ฅผ ์ฐธ๊ณ ํด์ ๊ณต๋ถํ๋ฉฐ ์์ฑํ์์ต๋๋ค>
https://data-panic.tistory.com/33
๐ ์ฝ๋ก๋ ํ์ง ์๋ฐฉ
- ํด์ธ์ ์ ํ์ง์์ ๋ํ ์๊ณ์ด(Time-Series) ๋ฐ์ดํฐ๋ฅผ ์ฌ์ฉํ์ฌ ์์ธก ๋ชจ๋ธ ๋ง๋ค๊ธฐ
- ๊ฐ๊น์ด ๋ฏธ๋์ ๋ฐ์ํ๋ ํด์ธ์ ์ ์ฌ๋ก๋ฅผ ์์ธก
- 14์ผ์ ๋ฏธ๋๊ฐ์ ์์ธกํ๋ ๊ฒ์ด ํ๋ก์ ํธ์ ๋ชฉํ
- ๋ชจ๋ธ๋ง์๋ PyTorch ๊ธฐ๋ฐ LSTM ๋ชจ๋ธ
๐ ์ฝ๋ ๋ฆฌ๋ทฐ
1๏ธโฃ Load libraries
import torch
import os
import numpy as np
import pandas as pd
from tqdm import tqdm
import seaborn as sns
from pylab import rcParams
import matplotlib.pyplot as plt
from matplotlib import rc
from sklearn.preprocessing import MinMaxScaler, StandardScaler
from sklearn.metrics import mean_squared_error
from pandas.plotting import register_matplotlib_converters
from torch import nn, optim
%matplotlib inline
%config InlineBackend.figure_format='retina'
sns.set(style='whitegrid', palette='muted', font_scale=1.2)
HAPPY_COLORS_PALETTE = ["#01BEFE", "#FFDD00", "#FF7D00", "#FF006D", "#93D30C", "#8F00FF"]
sns.set_palette(sns.color_palette(HAPPY_COLORS_PALETTE))
rcParams['figure.figsize'] = 14, 10
register_matplotlib_converters()
RANDOM_SEED = 42
np.random.seed(RANDOM_SEED)
torch.manual_seed(RANDOM_SEED)
import warnings
warnings.filterwarnings('ignore')
from matplotlib import font_manager, rc
2๏ธโฃ
df = pd.read_csv('final_0507.csv')
df.drop(["Unnamed: 0"], axis = 1, inplace = True)
df
- date setting
df.Date = pd.to_datetime(df.Date)
df.set_index('Date', inplace=True)
df
- ๋ณ์๋ช
- Date : ๋ ์ง(index)
- ๊ตญ๊ฐ์ฝ๋_conf : ํด๋น ๊ตญ๊ฐ์ ์ผ๋ณ ํ์ง์ ์
- ๊ตญ๊ฐ์ฝ๋_roam : ํด๋น ๊ตญ๊ฐ๋ก ๋ถํฐ ํ๊ตญ์ผ๋ก ๋ค์ด์จ ์ผ๋ณ ๋ก๋ฐ ์ด์ฉ์ ์
- KR : ๊ตญ๋ด ์ผ๋ณ ํ์ง์ ์ (์ง์ญ์ฌํ)
- news : ์ฝ๋ก๋ ๊ด๋ จ ํด์ธ ๋ด์ค ์ผ๋ณ ๊ฐฏ์
- covid_tr : 'covid' ํค์๋๋ก ๊ฒ์ํ ๊ตฌ๊ธ ํธ๋ ๋ ์ง์
- coro_tr : 'corona' ํค์๋๋ก ๊ฒ์ํ ๊ตฌ๊ธ ํธ๋ ๋ ์ง์
- target(ํด์ธ์ ์ ํ์ง์)
lag_col= list(df.columns)
lag_col
- ๋ชจ๋ ๋ณ์์ ์์ฐจ(LAG) ์์ฑ
- ๊ฐ ๋ณ์๋ค์ 3๊ฐ์ ์์ฐจ ๋ณ์ ๋ง๋ค๊ณ ์์ฑ์ผ๋ก ์ธํ NAN ๊ฐ์ ํต์งธ๋ก ๋ ๋ฆฌ๊ธฐ
lag_amount = 3
for col in lag_col:
for i in range(lag_amount):
df['{0}_lag{1}'.format(col,i+1)] = df['{}'.format(col)].shift(i+1)
df.dropna(inplace=True)
df
3๏ธโฃ Data exploration
print("total shape: {}".format(df.shape))
print("target feature shape: {}".format(df['target'].shape))
plt.figure(figsize=(25,5))
plt.plot(df['target'])
plt.xticks(rotation=90)
plt.title("Oversea Inflow Cofirmed")
plt.grid(axis='x')
4๏ธโฃ LSTM ๋ชจ๋ธ
X_cols = list(df.columns)
X_cols.remove('target')
- X, y์ ์ค์ผ์ผ๋ง
- Scikit-learn์ MinMaxScaler๋ฅผ ์ฌ์ฉ
- ์ค์ผ์ผ๋ ๋ฐ์ดํฐ๋ฅผ ์ถํ์ ๋ค์ inverse scale ํด์ฃผ๊ธฐ ์ํด X ๋ฐ์ดํฐ์ y๋ฐ์ดํฐ ๊ฐ๊ฐ ์ค์ผ์ผ๋ฌ๋ฅผ ๋ง๋ค์ด ์ ์ฉ
- ๊ทธ ํ์ train / test ์ ์ ๊ตฌ๋ถ
- lstm sequence๋ฅผ ๋ง๋ค์ด์ฃผ๊ธฐ ์ํด y๋ฐ์ดํฐ๋ฅผ flatten()ํ์ฌ ์ฐจ์์ ์ค์ด๊ธฐ
# MinMaxScaler ์ค์ผ์ผ๋ง
scaler = MinMaxScaler()
Xscaler = scaler.fit(X)
yscaler = scaler.fit(y.values.reshape(-1,1))
# ์ค์ผ์ผ๋ง ์ ์ฉ
X = Xscaler.fit_transform(X)
y = yscaler.fit_transform(y.values.reshape(-1,1))
# Train, Test set split
X_train, X_test = X[:-test_data_size], X[-test_data_size:]
y_train, y_test = y[:-test_data_size].flatten(), y[-test_data_size:].flatten()
print("train set : ", X_train.shape)
print("test set : ", X_test.shape)
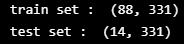
- LSTM ์ ์ํ ์ํ์ค ๋ฐ์ดํฐ ํ์ฑ ํจ์
- ๋ชจ๋ธ ์์ ๋ค์ด๊ฐ ๋ฐ์ดํฐ๋ฅผ ์ํ์ค ํํ๋ก ๋ง๋ค์ด ์ฃผ๊ธฐ ์ํ ํจ์
def create_sequences1(array, seq_length):
res = []
if seq_length == 1:
for i in range(len(array)):
tmp=array[i:(i+seq_length)]
res.append(tmp)
else:
for i in range(len(array)-seq_length-1):
tmp = array[i:(i+seq_length)]
res.append(tmp)
return res
โ ํจ์๋ฅผ ์ฌ์ฉํ์ฌ ๋ฐ์ดํฐ๋ฅผ ์ํ์คํํ๋ก ๋ง๋ฆ
โ 5๊ฐ ์ฉ ํ ์ํ์ค๋ก ๋ฌถ์์ ๊ฒฝ์ฐX_train์ ๋ณด๋ฉด ํ 1๊ฐ์ array์ 5๊ฐ์ ๋ฐ์ดํฐ๊ฐ ๋ค์ด๊ฐ ์์
= 1์ 22์ผ ๋ถํฐ 1์ 26์ผ๊น์ง์ X ๋ฐ์ดํฐ๊ฐ ํ๋๋ก ๋ฌถ์ฌ์ ๋ชจ๋ธ๋ก ๋ค์ด๊ฐ๋ ๊ตฌ์กฐ
โ ์ํ์ค ํํ๋ก ๋ง๋๋ ์ด์ ๋ ์๊ณ์ด ๋ฐ์ดํฐ์ ์์๋ฅผ ํ์ต์ํค๊ธฐ ์ํจ
seq_length = 5 X_train = create_sequences1(X_train, seq_length) y_train = create_sequences1(y_train, seq_length) X_test = create_sequences1(X_test, seq_length) y_test = create_sequences1(y_test, seq_length) X_train[:3]
-
์ํ์ค๋ฅผ ๋ง๋ค์ง ์๊ณ ์ฌ์ฉํ๋ ค๋ฉด seq_length๋ฅผ 1
-
๋ชฉํํ๋ ๊ฒ์ 14์ผ ์์ธกํ๋ ๊ฒ
-
์ํ์ค๋ฅผ ๋ง๋ฆ์ผ๋ก์จ ๋ฐ์ดํฐ ๋ํ ๋ ํ์
-
๊ฐ์ง๊ณ ์๋ ๋ฐ์ดํฐ๋ ํ์ ๋์ด์๊ธฐ ๋๋ฌธ์ ์ํ์ค๋ฅผ ๋ง๋ค์ง ์๊ณ ์ฌ์ฉ
-
seq_length = 1
X_train = create_sequences1(X_train, seq_length)
y_train = create_sequences1(y_train, seq_length)
X_test = create_sequences1(X_test, seq_length)
y_test = create_sequences1(y_test, seq_length)
X_train[:3]
- PyTorch ๋ชจ๋ธ์ ๋ฐ์ดํฐ๋ฅผ ์ฌ๋ฆฌ๊ธฐ ์ํด torch.tensor๋ก ๋ณํ
# numpy๋ฅผ tensor๋ก ๋ณํ
X_train = torch.tensor(X_train).float()
y_train = torch.tensor(y_train).float()
X_test = torch.tensor(X_test).float()
y_test = torch.tensor(y_test).float()
print("X_train :",(X_train.shape))
print("X_test :",(X_test.shape))
print("y_train :",(y_train.shape))
print("y_test :",(y_test.shape))
5๏ธโฃ LSTM ๋ชจ๋ธ ์์ฑ
- LSTM๊ณผ Linear๋ก ๊ตฌ์ฑ
- num_layers๋ก ๋ ์ด์ด์ธต์ ๊ฐฏ์๋ฅผ ์ค์ ํ ์ ์๋๋ก ํจ
- ๋ฐ์ดํฐ๊ฐ ์ ๊ณ ๋ฅ๋ฌ๋ ๋ชจ๋ธ์ ํฌ๊ฑฐ๋ ๊น์ง ์์ผ๋ฏ๋ก dropout์ ๋ฐ๋ก ์ฃผ์ง ์์
# ๋ชจ๋ธ Clss ์์ฑ
class CoronaVirusPredictor(nn.Module):
def __init__(self, n_features, n_hidden, seq_len, n_layers=2):
super(CoronaVirusPredictor, self).__init__()
self.n_hidden = n_hidden
self.seq_len = seq_len
self.n_layers = n_layers
self.lstm = nn.LSTM(
input_size = n_features,
hidden_size = n_hidden,
num_layers = n_layers,
#dropout=0.1
)
self.linear = nn.Linear(in_features=n_hidden, out_features=1)
def reset_hidden_state(self):
self.hidden = (
torch.zeros(self.n_layers, self.seq_len, self.n_hidden),
torch.zeros(self.n_layers, self.seq_len, self.n_hidden))
def forward(self, sequences):
lstm_out, self.hidden = self.lstm(sequences.view(len(sequences), self.seq_len, -1), self.hidden)
last_time_step = lstm_out.view(self.seq_len, len(sequences), self.n_hidden)[-1]
y_pred = self.linear(last_time_step)
return y_pred
6๏ธโฃ ๋ชจ๋ธ ํ๋ จ
- epoch๊ณผ learning rate๋ฅผ ํ๋ผ๋ฏธํฐ๋ก ์ค์ ํ ์ ์๊ฒ ํจ
- loss function์ผ๋ก๋ MSELoss
- optimizer๋ก Adam
- optimizer์ weight_decay๋ฅผ ์ค์
- 10 epoch ๋ง๋ค train๊ณผ test์ loss๋ฅผ ์ถ๋ ฅ
def train_model(model, train_data, train_labels, test_data=None, test_labels=None, num_epochs=250, lr=1e-3):
loss_fn = torch.nn.MSELoss()
optimiser = torch.optim.Adam(model.parameters(), lr=lr, weight_decay=1e-4)
num_epochs = num_epochs
train_hist = np.zeros(num_epochs)
test_hist = np.zeros(num_epochs)
for t in range(num_epochs):
model.reset_hidden_state()
y_pred = model(X_train)
loss = loss_fn(y_pred.float(), y_train)
if test_data is not None:
with torch.no_grad():
y_test_pred = model(X_test)
test_loss = loss_fn(y_test_pred.float(), y_test)
test_hist[t] = test_loss.item()
if t % 10 == 0:
print(f'Epoch {t} train loss: {round(loss.item(),4)} test loss: {round(test_loss.item(),4)}')
elif t % 10 == 0:
print(f'Epoch {t} train loss: {loss.item()}')
train_hist[t] = loss.item()
optimiser.zero_grad()
loss.backward()
optimiser.step()
return model.eval(), train_hist, test_hist
# ํ์ดํผํ๋ผ๋ฏธํฐ
n_features=X_train.shape[-1]
n_hidden=64
n_layers=4
lr=1e-4
num_epochs=200
# Training Model
model = CoronaVirusPredictor(n_features=n_features, n_hidden=n_hidden, seq_len=seq_length, n_layers=n_layers)
model, train_hist, test_hist = train_model(model, X_train, y_train, X_test, y_test, num_epochs=num_epochs, lr=lr)
-
ํ์ต ๊ฒฐ๊ณผ
-
loss๊ฐ ์๋ ดํ๋ ๋ชจ์ต์ ๋ณด๋ฉด ์๋นํ ๋น์ ์์ ์ธ ๊ฒ
-
์ฐ์ train๋ณด๋ค test์ loss๊ฐ ๋ ๋ฎ์
-
1) train data๊ฐ ๋๋ฌด ์ด๋ ต๊ฑฐ๋ test data๊ฐ ๋๋ฌด ์ฌ์ธ ๊ฒฝ์ฐ์ train loss ๋ณด๋ค test loss๊ฐ ๋ฎ๊ฒ ๋์ฌ ์ ์์
-
2) 100์ฌ๊ฐ ์ ๋์ ๋ฐ์ดํฐ๋ฅผ ๊ฐ์ง๊ณ ๋ฅ๋ฌ๋ ๋ชจ๋ธ์ ๋๋ ธ์ผ๋ ์ฌ์ค ์ ์์ ์ธ ํ์ต์ ์๋ -> ๋ฐ์ดํฐ์ ์๊ฐ ๋๋ฌด ์ ์
-
-
# plotting Loss
plt.plot(train_hist, label="Training loss")
plt.plot(test_hist, label="Test loss")
plt.title('n_features:{0}, n_hidden:{1}, n_layers:{2}, lr:{3}, seq_length:{4}, num_epochs:{5}'.format(n_features,n_hidden,n_layers,lr,seq_length,num_epochs))
plt.legend()
7๏ธโฃ ์ผ์ผ ์ผ์ด์ค ์์ธก
with torch.no_grad():
preds = []
for i in range(len(X_test)):
test_seq = X_test[i:i+1]
y_test_pred = model(test_seq)
pred = torch.flatten(y_test_pred).item()
preds.append(pred)
new_seq = test_seq.numpy().flatten()
new_seq = np.append(new_seq, pred)
new_seq = new_seq[1:]
test_seq = torch.as_tensor(new_seq).view(n_features, seq_length, 1).float()
-
X_test ๊ฐ์ ๋ชจ๋ธ์ ๋ฃ์ด ์์ธก๊ฐ preds๋ฅผ ์ฐ์ถ
-
์์์ ์ ๊ฒฐ๊ณผ๊ฐ๋ค์ด ๋์ค๋๋ฐ ์ด๊ฑด ์์์ ๋ฐ์ดํฐ๋ฅผ ์ค์ผ์ผ๋ง ํด์คฌ๊ธฐ ๋๋ฌธ
# Prediction value ์ญ๋ณํ
pred_values = yscaler.inverse_transform(np.array(preds).reshape(-1,1))
- X_test ๊ฐ์ ๋ชจ๋ธ์ ๋ฃ์ด ์์ธก๊ฐ pr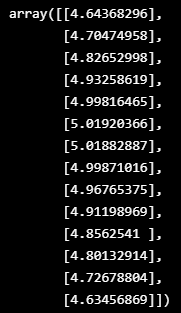
eds๋ฅผ ์ฐ์ถ
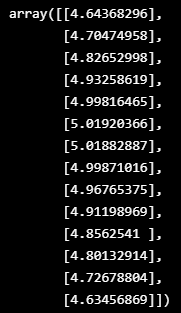
- ์์์ ์ ๊ฒฐ๊ณผ๊ฐ๋ค์ด ๋์ค๋๋ฐ ์ด๊ฑด ์์์ ๋ฐ์ดํฐ๋ฅผ ์ค์ผ์ผ๋ง ํด์คฌ๊ธฐ ๋๋ฌธ
pred_values_ceiled = list(pred_values.flatten())
# True value ์ญ๋ณํ
true_values = yscaler.inverse_transform(y_test)[:, [-1]]
# ์ค์ ๊ฐ ์์ธก๊ณผ ๋ฐ์ดํฐ ํ๋ ์ ์์ฑ
score_table = pd.DataFrame({'True':true_values.flatten(),
'Pred':pred_values_ceiled})
-
์ค์ y๊ฐ 'True'์ ๋ชจ๋ธ๋ก ๋ถํฐ ๋์จ ์์ธก๊ฐ 'Pred'๋ก ๊ตฌ์ฑ๋ score_table
-
4์ 22์ผ ๋ถํฐ 5์5์ผ๊น์ง์ ์ค์ ๊ฐ๊ณผ ์์ธก๊ฐ
- MSE์ RMSE๋ฅผ ์์ฑ
- score๋ ์ค์ ๊ฐ๊ณผ ์์ธก๊ฐ์ ์ฐจ์ด๊ฐ ์์ ์๋ก 100์ ๊ฐ๊น์์ง๋ ์ ์
# validation score
MSE = mean_squared_error(score_table['True'], score_table['Pred'])
RMSE = np.sqrt(MSE)
score = 100*(1-(((score_table['Pred'] -score_table['True'])**2).sum())/((score_table['True']**2).sum()))
print("MSE : {0}, RMSE : {1}, SCORE : {2}".format(MSE, RMSE, score))
- ๋ ธ๋์ ์ด ์ค์ ๊ฐ์ด๊ณ ๋นจ๊ฐ์ ์ด ์์ธก๊ฐ
- ์ค์ ํด์ธ์ ์ ํ์ง์์ ๊ฒฝ์ฐ์๋ ์ผ์ผ๋ณ ๊ตด๊ณก๋ค์ด ์์
plt.figure(figsize=(10,5))
plt.plot(range(y_train.__len__()),yscaler.inverse_transform(y_train)[:, [-1]])
plt.plot(range(y_train.__len__(), y_train.__len__()+y_test.__len__()),true_values, label='Real')
plt.plot(range(y_train.__len__(), y_train.__len__()+y_test.__len__()),pred_values_ceiled, label='Pred')
#plt.xlim(70)
plt.legend()
- PyTorch์ ๋ชจ๋ธ ํ์ฅ์ ํ์์ธ .pth๋ก ์ ์ฅ
- ๋ชจ๋ธ์ ํ์ผ๋ช ์ ์ฌ์ฉํ ํ๋ผ๋ฏธํฐ์ ์ ์๋ฅผ ๋ฃ์ด ์ด๋ค ๋ชจ๋ธ์ด์๋์ง ๊ตฌ๋ถ ๊ฐ๋ฅ
# ๋ชจ๋ธ ์ ์ฅ
PATH = './{6}_n_features_{0}_n_hidden_{1}_n_layers_{2}_lr_{3}_seq_length_{4}_num_epochs_{5}.pth'.format(n_features,n_hidden,n_layers,lr,seq_length,num_epochs, score.round(2))
torch.save(model, PATH)
8๏ธโฃ ์ ์ฒด ๋ฐ์ดํฐ๋ฅผ ์ฌ์ฉํ์ฌ ๋ฏธ๋ ์์ธก
- ๋์ผํ๊ฒ ๋ฐ์ดํฐ๋ฅผ ์ ์ฒ๋ฆฌ
- Train, Test๋ฅผ ๋๋๋ ๊ฒ์ด ์๋ ์ ์ฒด ๋ฐ์ดํฐ๋ฅผ ์ฌ์ฉ
X_all = df[X_cols]
y_all = df['target']
# MinMaxScaler ์ค์ผ์ผ๋ง
scaler = MinMaxScaler()
# X scaler
Xscaler = scaler.fit(X_all)
# Y scaler
yscaler = scaler.fit(y_all.values.reshape(-1,1))
# ์ค์ผ์ผ๋ง ์ ์ฉ
X_all = Xscaler.fit_transform(X_all)
y_all = yscaler.fit_transform(y_all.values.reshape(-1,1))
y_all = y_all.flatten()
print("X_all : ", X_all.shape)
print("y_all : ", y_all.shape)
X_all = create_sequences1(X_all, seq_length)
y_all = create_sequences1(y_all, seq_length)
X_all = torch.from_numpy(np.array(X_all)).float()
y_all = torch.from_numpy(np.array(y_all)).float()
- DAYS_TO_PREDICT๋ ์์ธกํ ๋ ์ง์ ์
- 14์ผ์ ์์ธกํ๋ ๊ฒ์ด ๋ชฉํ์๊ธฐ ๋๋ฌธ์ 14๋ก ์ค์
DAYS_TO_PREDICT = 14
with torch.no_grad():
test_seq = X_all[:1]
preds = []
for _ in range(DAYS_TO_PREDICT):
y_test_pred = model(test_seq)
pred = torch.flatten(y_test_pred).item()
preds.append(pred)
new_seq = test_seq.numpy().flatten()
new_seq = np.append(new_seq, [pred])
new_seq = new_seq[1:]
pred_values = yscaler.inverse_transform(np.array(preds).reshape(-1,1))
- 14์ผ ์น์ ๋ฏธ๋ ์์ธก๊ฐ
- 4๋ช ์์ 1๋ช ๊น์ง ๋จ์ด์ง
- ๋ชจ๋ธ์ด ์ค์ ๋ก ํด์ธ์ ์ ํ์ง์์ ๊ฐ์๋ฅผ ํ์ต์ ํ๊ฑด์ง ์๋๋ฉด ๋ฑํ ์์ธก์ ๋ํ ๊ทผ๊ฑฐ๋ ํ์ด ์์ด์ ๋จ์ด์ง๊ฑฐ๋ผ๊ณ ์์ธกํ๊ฑด์ง๋ ์ ์๊ฐ ์์
import math
pred_values_ceiled = list(pred_values.flatten())
predicted_cases=pred_values_ceiled
predicted_cases
predicted_index = pd.date_range(
start=df.index[-1],
periods=DAYS_TO_PREDICT + 1,
closed='right'
)
predicted_index = pd.to_datetime(predicted_index, format='%Y%m%d')
predicted_cases = pd.Series(
data=predicted_cases,
index=predicted_index
)
plt.plot(predicted_cases, label='Predicted Daily Cases')
plt.legend();
preds_ = pd.DataFrame(predicted_cases)
df.index = pd.to_datetime(df.index)
plt.figure(figsize=(25,5))
plt.plot(df['target'].astype(int), label='Historical Daily Cases')
plt.plot(preds_, label='Predicted Daily Cases')
plt.xticks(rotation=90)
plt.title("Oversea Inflow Cofirmed")
plt.grid(axis='x')
plt.legend()
728x90
๋ฐ์ํ
'๐ฉโ๐ป ์ธ๊ณต์ง๋ฅ (ML & DL) > Serial Data' ์นดํ ๊ณ ๋ฆฌ์ ๋ค๋ฅธ ๊ธ
CNN-LSTM ์ผ๋ก ์๊ณ์ด ๋ถ์ํ๊ธฐ (0) | 2022.11.04 |
---|---|
Serial Data ์ฅ์ ์์ธก/๊ฐ์ง LSTM & Conv ๋ชจ๋ธ (0) | 2022.10.28 |
[FuncAnimation] 4. Mongo DB์ ์๊ณ์ด ๋ฐ์ดํฐ ์ ์ฅํ๊ธฐ (2) (0) | 2022.10.25 |
[FuncAnimation] 3. Mongo DB์ ์๊ณ์ด ๋ฐ์ดํฐ ์ ์ฅํ๊ธฐ (1) (0) | 2022.10.24 |
[FuncAnimation] 2. ๋จ์ผ๋ณ๋ ๊ทธ๋ํ๋ฅผ ๋ง๋ค์ด์ GUI๋ก ์๊ฐํํ๊ธฐ (0) | 2022.10.24 |
Comments