๋ชฉ๋ก๐ฉ๐ป ์ธ๊ณต์ง๋ฅ (ML & DL) (100)
๐ ๊ณต๋ถํ๋ ์ง์ง์ํ์นด๋ ์ฒ์์ด์ง?
๐ Train Set Train set์ ์จ์ ํ ๋ชจ๋ธ์ ํ์ต๋ง์ ์ํด์ ์ฌ์ฉ parameter๋ feature๋ฑ์ ์์ ํด์ ๋ชจ๋ธ์ ์ฑ๋ฅ์ ๋์ด๋ ์์ ์ ๊ฑฐ์น ๋ ์ฌ์ฉ ๐Validation Set Validation set๊ณผ test set์ ๋ชจ๋ธ์ ํ์ต์ ์ง์ ์ ์ผ๋ก ๊ด์ฌํ์ง ์์ ํ์ต์ด ๋๋ ๋ชจ๋ธ์ ์ ์ฉ์์ผ test set์ ์ด์ฉํ ๋ชจ๋ธ์ ํ๊ฐ๋ก ๋์ด๊ฐ๊ธฐ ์ด์ ์ ์ต์ข ์ ์ผ๋ก ๋ชจ๋ธ์ fine tuningํ๋๋ฐ์ ์ฌ์ฉ ๋ง๋ ๋ชจ๋ธ์ด test set ์์, ๊ทธ๋ฆฌ๊ณ ์ค์ ๋ก ๋ฐฐํฌ ๋์์๋ ๋์ ์ฑ๋ฅ์ ๋ผ ์ ์๋๋ก ๋ง๋ค์ด ์ค ๐Test Set Test set์ ์ต์ข ์ ์ผ๋ก ์ฐ๋ฆฌ๊ฐ ๋ง๋ ๋ชจ๋ธ์ ์ฑ๋ฅ์ ํ๊ฐํ๊ธฐ ์ํ ๋ฐ์ดํฐ์ ๋ชจ๋ธ์ ํ์ต์๋ ์ ํ ์ฌ์ฉ๋์ง ์์ ๋ชจ๋ธ์ด ๋ฐฐํฌ๋ ์ดํ์ ์ค์ฌ์ฉ ๋์์๋ ๋ชจ๋ธ์ด ์ผ๋ง๋ ์ข์ ์ฑ๋ฅ์..
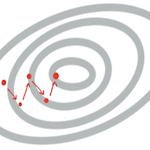
batch_size : ๋งค ํ๋ จ ๋จ๊ณ๋ง๋ค ํ์ตํ ๋ฐ์ดํฐ์ ํฌ๊ธฐ๋ฅผ ๊ฒฐ์ epoch : ๋ฐฐ์น ์ฌ์ด์ฆ๋ก ๋ถํ ๋ ํ๋ จ ๋ฐ์ดํฐ ์ ์ฒด๋ฅผ 1ํ ํ์ตํ๋ ํ์๋ฅผ ๋ช ๋ฒ ๋ฐ๋ณตํ ์ง learning rate : ๋ชจ๋ธ์ weight์ด ์ ๋ฐ์ดํธ ๋ ๋๋ง๋ค ์์ ์ค๋ฅ์ ๋ํ ์๋ต์ผ๋ก ๋ชจ๋ธ์ ์กฐ์ ๐ Learning rate ํด ๋ ํ ๋ฒ์ step์์ ํ์ต์ด ํฌ๊ฒ ์งํ ๋ณดํญ์ด ํฌ๊ธฐ ๋๋ฌธ์ ์ข ๋ ๋นจ๋ฆฌ ์๋ ด ์ค๋ฒ์์ด ์ฌํ๊ฒ ์ผ์ด๋ loss๊ฐ ์ ํ ์ค์ง ์์ ๐ Learning rate ์์ ๋ step ๋ณดํญ์ด ์์ ์กฐ๊ธ์ฉ ํ์ต ์์ ๋ณดํญ ๋๋ฌธ์ local minima์ ๋น ์ง ๐ฆ batch_size ํด ๋ ํ ๋ฒ ํ์ตํ ๋ ๋ง์ ๋ฐ์ดํฐ๋ก ํ์ต batch๊ฐ ํฌ๋ฉด ๊ณ์ฐ๋๋ loss๊ฐ์ ํธ์ฐจ๊ฐ ์์ ๊ณผ์ ํฉ ์ํ ๐ฆ batch_size ์..
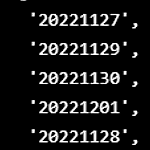
๐ฆ ์ ์ฒ๋ฆฌ ํ๊ธฐ ์ ์ ๋ฐ์ดํฐ ๊ตฌ๊ฒฝ~ ๐ธ1. ์ฌ๋ฌ ํด๋ ์์ CSV ํ์ผ ํฉ์น๊ธฐ import numpy as np # linear algebra import pandas as pd # data processing, CSV file I/O (e.g. pd.read_csv) import matplotlib.pyplot as plt import math as math %matplotlib inline import pickle import os # Load the data forder_list = os.listdir("./DATA") forder_list csv1 = [] csv2 = [] csv3 = [] csv4 = [] csv5 = [] csv6 = [] for forder in forder_list : p..
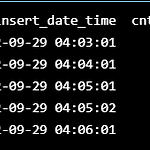
https://keras.io/examples/timeseries/timeseries_anomaly_detection/#introduction Keras documentation: Timeseries anomaly detection using an Autoencoder Timeseries anomaly detection using an Autoencoder Author: pavithrasv Date created: 2020/05/31 Last modified: 2020/05/31 Description: Detect anomalies in a timeseries using an Autoencoder. View in Colab • GitHub source Introduction This script demo..
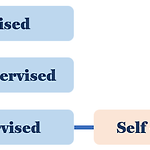
https://daeun-computer-uneasy.tistory.com/37 [CV] Self-supervised learning(์๊ธฐ์ฃผ๋ํ์ต)๊ณผ Contrastive learning - ์ค์ค๋ก ํ์ตํ๋ ์๊ณ ๋ฆฌ์ฆ ์ค๋์ Self-supervised learning(์๊ธฐ์ฃผ๋ํ์ต)๊ณผ ์ฃผ๋ ํ์ต ๋ฐฉ๋ฒ์ธ Contrastive learning์ ๋ํด ํฌ์คํ ํ๊ฒ ์ต๋๋ค. ๋จผ์ Self supervised learning์ด ์ ํ์ํ์ง๋ถํฐ ์ดํด๋ณด๊ฒ ์ต๋๋ค. Self-supervised learning์ ํ์ daeun-computer-uneasy.tistory.com ๐ฐ ์๊ธฐ์ฃผ๋ํ์ต(Self-supervised learning) ๋ฅ๋ฌ๋ ํ์ต์๋ ์ถฉ๋ถํ ์์ง์ ๋ฐ์ดํฐ๊ฐ ํ์ ์ด๋ฌํ ๋ฐ์ดํฐ๋ค์ ์ง๋ํ์ต์ ์ํด์๋ ๋ผ๋ฒจ๋ง..
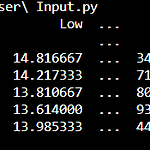
https://pythonprogramming.net/dynamic-data-visualization-application-dash-python-tutorial/ Python Programming Tutorials Dynamic Graph based on User Input - Data Visualization GUIs with Dash and Python p.3 Welcome to part three of the web-based data visualization with Dash tutorial series. Up to this point, we've learned how to make a simple graph and how to dynamically upda pythonprogramming.net..
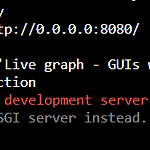
https://pythonprogramming.net/live-graphs-data-visualization-application-dash-python-tutorial/ Python Programming Tutorials Live Graphs - Data Visualization GUIs with Dash and Python p.4 Welcome to part four of the web-based data visualization with Dash tutorial series. In this tutorial, we're going to be create live updating graphs with Dash and Python. Live graphs can be usef pythonprogramming..
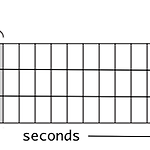
https://data-newbie.tistory.com/31 ๋ชจ๋ธํ๊ฐ์ ์ฑ๋ฅํ๊ฐ _๋ฏธ์์ฑ ๋์์ด ๋์ จ๋ค๋ฉด, ๊ด๊ณ ํ๋ฒ๋ง ๋๋ฌ์ฃผ์ธ์. ๋ธ๋ก๊ทธ ๊ด๋ฆฌ์ ํฐ ํ์ด ๋ฉ๋๋ค ^^ ์ง๋์ ๋น์ง๋ ๋ค์ํ ๋จธ์ ๋ฌ๋ ์๊ณ ๋ฆฌ์ฆ ์ดํด๋ด -> ๋ชจ๋ธ ํ๊ฐ์ ๋งค๊ฐ๋ณ์ ์ ํ์ ๋ํด ์์๋ณด์ ๋น์ง๋ ํ์ต์ ์ data-newbie.tistory.com ๐ฅ 1D CNN (1 Dimensional Convolution Neural Network) CNN ๋ชจ๋ธ์ 1D, 2D, 3D๋ก ๋๋๋๋ฐ, ์ผ๋ฐ์ ์ธ CNN์ ๋ณดํต ์ด๋ฏธ์ง ๋ถ๋ฅ์ ์ฌ์ฉ๋๋ 2D๋ฅผ ํต์นญ D๋ ์ฐจ์์ ๋ปํ๋ dimensional์ ์ฝ์๋ก, ์ธํ ๋ฐ์ดํฐ ํํ์ ๋ฐ๋ผ 1D, 2D, 3..